The development of AI has transformed sectors by spurring automation and innovation. Examine its development, uses, and effects.
What Is Artificial Intelligence?
The simulation of human intelligence in machines that are designed to think, learn, and make decisions is known as artificial intelligence. Machine learning, natural language processing, robotics, and computer vision are some subfields that fall under the umbrella of artificial intelligence. At its core, AI aims to enable machines to perform tasks typically requiring human intelligence, such as problem-solving, reasoning, and understanding language. By analyzing data, recognizing trends, and generating predictions, AI has become a vital tool across industries, from healthcare to finance.
As technology advances, the definition of artificial intelligence (AI) has changed over time. Experts classify AI into three categories: narrow AI, general AI, and super-intelligent AI. Narrow AI performs specific tasks, like recommendation algorithms or virtual assistants; general AI, which is still a theoretical concept, aims to replicate human-like intelligence and adaptability; and super-intelligent AI, which is frequently a topic of conjecture, would outperform human intelligence in almost every field. It is important to comprehend these differences as we examine the history and evolution of AI.
AI is also characterized by its methodologies. Furthermore, data science, psychology, linguistics, and neurology are now all involved in the multidisciplinary field of artificial intelligence. These disciplines’ cooperation has sped up the development of AI, increasing its resilience and suitability for solving real-world problems . As technology advances, the development of AI continues to play a crucial role in enhancing human capacities and decision-making keeps growing.
The History of Artificial Intelligence
The history of artificial intelligence can be traced back to ancient civilizations, where stories and myths depicted human-made intelligent beings. These early examples include the Greek myth of Talos, a massive bronze automaton, and ancient Chinese mechanical inventions. These concepts mirrored humanity’s fascination with creating artificial beings and foreshadowed current efforts to create intelligent machines.
In the modern era, pioneers like Alan Turing laid the groundwork for artificial intelligence with his 1950 paper “Computing Machinery and Intelligence,” which examined the possibility of machines displaying intelligent behavior. Turing’s idea of the Turing Test is still used to assess machine intelligence, and early computers were developed in the 1940s and 1950s that were able to perform calculations far beyond human capacity. These machines provided a platform for investigating the potential of AI.
The development of AI symbolic logic, cybernetics, and computational theories. For example, Norbert Wiener’s work on feedback loops and Claude Shannon’s information theory shed light on how machines process and communicate information. These early advances laid the groundwork for formalizing AI as a separate field of research.
Groundwork for AI
The groundwork for AI was established through interdisciplinary efforts involving mathematics, computer science, neuroscience, and cognitive psychology. Researchers aimed to understand and dissect human intelligence into its constituent processes, reasoning, perception, and learning. A wide range of disciplines, including biology, philosophy, and linguistics, inspired this method.
The Logic Theorist, created in 1956 by Allen Newell and Herbert A. Simon, was among the first artificial intelligence programs. The program, which creators designed to establish mathematical theorems, showed that machines could simulate human problem-solving. John McCarthy’s creation of the Lisp programming language, which served as a foundation for the advancement of artificial intelligence because of its proficiency with processing recursive data structures and manipulating symbols, was another significant event.
Inspired by the structure of the human brain, researchers also explored neural networks during this time. The development of AI benefited from early models like the Perceptron created by Frank Rosenblatt, which attempted to mimic biological systems’ learning processes; however, these attempts were hindered by a lack of hardware and theoretical limitations, but they set the groundwork for later advances in machine learning.
During the Cold War, government and military funding for AI research further strengthened the field, with projects aimed at creating intelligent systems for strategic purposes highlighting AI’s capacity to solve complex problems. During this time, foundational theories and tools emerged that still influence AI research today.
Birth of AI
AI as a field of study officially began in 1956 at the Dartmouth Conference. Where leading researchers gathered to discuss the potential for creating intelligent machines. The symposium, which was organized by Claude Shannon, Nathaniel Rochester, John McCarthy, and Marvin Minsky, was a turning point in the history of artificial intelligence. Participants listed lofty objectives, such as creating software that could mimic logic, play games, and comprehend language.
The Dartmouth Conference’s confidence advanced AI quickly. Researchers created early programs that were able to play checkers, solve algebraic equations, and prove logical claims. Arthur Samuel demonstrated one of the first instances of machine learning with his checkers software, which not only played the game but also enhanced its performance through self-learning.
Another significant achievement was the creation of ELIZA, a natural language processing program developed by Joseph Weizenbaum. By simulating human speech, ELIZA showed that machines are capable of meaningfully interacting with people. These achievements stoked interest in and funding for AI research, opening the door for more investigation.
There was a sense of boundless potential in the early days of artificial intelligence. Within a few decades, researchers thought, human-level intelligence could be attained. This hope fueled large investments in AI initiatives, setting the stage for further advancements.
AI Maturation
The 1960s and 1970s were characterized by the maturation of AI as researchers developed more sophisticated algorithms and practical applications. Expert systems, which use rule-based reasoning to solve issues in certain domains, were a significant innovation. AI’s potential in domains like chemistry and medicine was demonstrated by programs like DENDRAL and MYCIN, which performed some tasks better than human experts.
AI researchers have branched out into robotics, where they created machines to do everything from product assembly to space exploration. While advances in computer vision allowed robots to perceive and navigate their surroundings. The advent of heuristic search techniques enabled machines to solve complex problems more effectively. Notwithstanding these advancements, the high expenses of computational resources and the inflexibility of rule-based systems constrained the development of AI.
Researchers investigated neural networks and machine learning at the same time. Although early neural network models encountered theoretical and practical difficulties, they offered a conceptual framework for subsequent advances.
Researchers studying human cognition have significantly influenced the development of AI by modeling how machines make decisions, learn, and remember. As AI evolved, universities and research institutions sprang up, dedicated to exploring this exciting field. Collaboration between government agencies, industry leaders, and educational institutions played a crucial role in driving innovation forward.
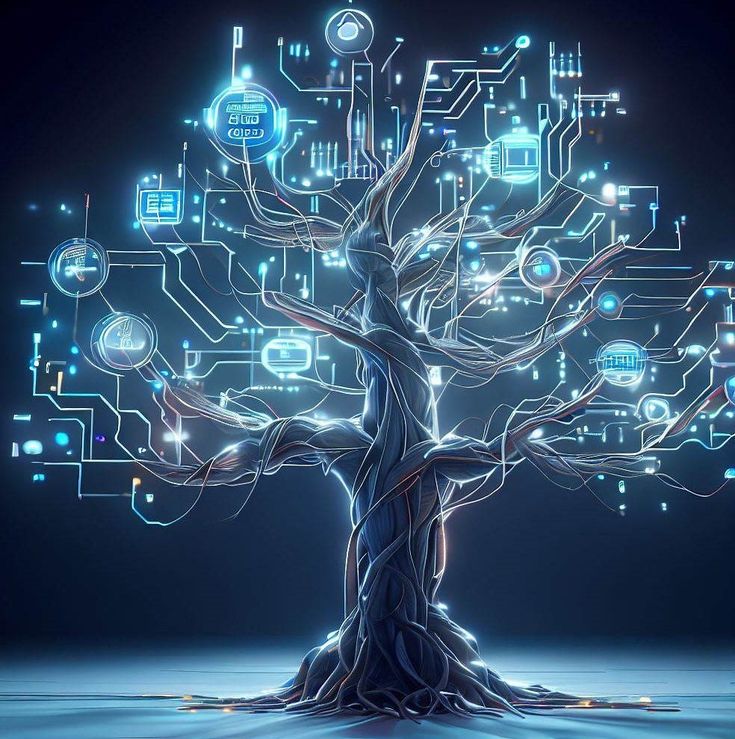
AI Winter
There were AI winters in the late 1970s and late 1980s, which were characterized by a lack of funding and distrust. Unfulfilled expectations and technological constraints throughout these times weakened faith in AI’s promise. Expert systems, for example, frequently failed to adjust to novel situations, underscoring the need for more adaptable and reliable strategies.
AI research went on in spite of these obstacles, but more slowly. Scholars turned their attention to investigating alternative paradigms and resolving the shortcomings of current systems. AI winters also taught us how important it is to have reasonable expectations and match research objectives with real-world uses.
AI Boom
Due to developments in hardware, algorithms, and funding, the 1980s saw a boom in artificial intelligence. The widespread adoption of expert systems in sectors including healthcare, manufacturing, and finance demonstrated the commercial viability of AI. AI research came from governments and businesses, which accelerated the development of AI, resulting in the creation of specialized research facilities and educational initiatives.
With the creation of techniques like backpropagation, which allowed neural networks to learn from data more efficiently, machine learning gained popularity during this time. Additionally, robotics advanced, as seen by devices like Honda’s ASIMO, which can walk, recognize faces, and react to voice directions.
The AI boom brought some challenges. Early systems depended heavily on hand-coded rules, which made them fragile and tough to expand. The development of AI was also hindered by the difficulty people faced in accepting these systems, as setting them up and maintaining them was quite expensive. These issues eventually led to a decline in interest and funding, kicking off what we now call the AI winter.
AI Agents
The rise of intelligent agents, systems that can see their surroundings, make decisions, and act to accomplish particular objectives , was the primary driver of artificial intelligence’s comeback in the 1990s. Developments in machine learning, robotics, and data analytics have made it possible to create autonomous systems like virtual assistants, recommendation engines, and self-driving cars.
The expansion of the internet and the accessibility of huge datasets drove this resurgence. While developments in robotics made it possible for engineers to create drones and automated production systems, machine learning techniques drove applications that ranged from search engines to fraud detection systems. The development of AI further advanced these technologies, as AI agents changed sectors and increased production, becoming a necessary part of daily life.
Additionally, AI agents brought with them additional difficulties, such as resolving biases in decision-making, controlling privacy concerns, and guaranteeing their ethical use. Researchers and policymakers are still developing frameworks for the appropriate deployment of AI.
The Rise of Machine Learning
Machine learning (ML) stands out as a key component of modern AI, enabling systems to learn from data, adapt to new information, and improve over time. These models dive into large datasets to uncover patterns and make predictions. This approach differs from traditional programming, where developers write explicit rules. Thanks to this shift in perspective, AI has evolved significantly and found applications across various fields
With the introduction of more potent algorithms and more processing power in the 1990s, machine learning started to gain traction. Building intelligent systems now relies heavily on supervised learning, unsupervised learning, and reinforcement learning. By using labeled data to train models, supervised learning makes it possible for them to produce precise predictions. While reinforcement learning enables machines to learn by trial and error and optimize their behavior based on feedback, unsupervised learning finds hidden patterns in data without explicit labels.
Deep learning, a subset of machine learning, has further enhanced the capabilities of AI. By utilizing multi-layered artificial neural networks, deep learning models effectively process complicated data, including text, audio, and images. AI has become more adaptable and efficient because of advances in deep learning, which have fueled technologies like speech synthesis, facial recognition, and natural language comprehension.
Industries have also changed due to the emergence of machine learning. Healthcare professionals use ML algorithms to assist with disease diagnosis and predict patient outcomes. In the financial industry, companies improve investment plans and identify fraudulent transactions using these algorithms. Machine learning (ML) supports autonomous vehicles in transportation, allowing them to maneuver through challenging terrain. As data volumes increase, machine learning continues to play a key role in the development of AI, shaping its impact on society.
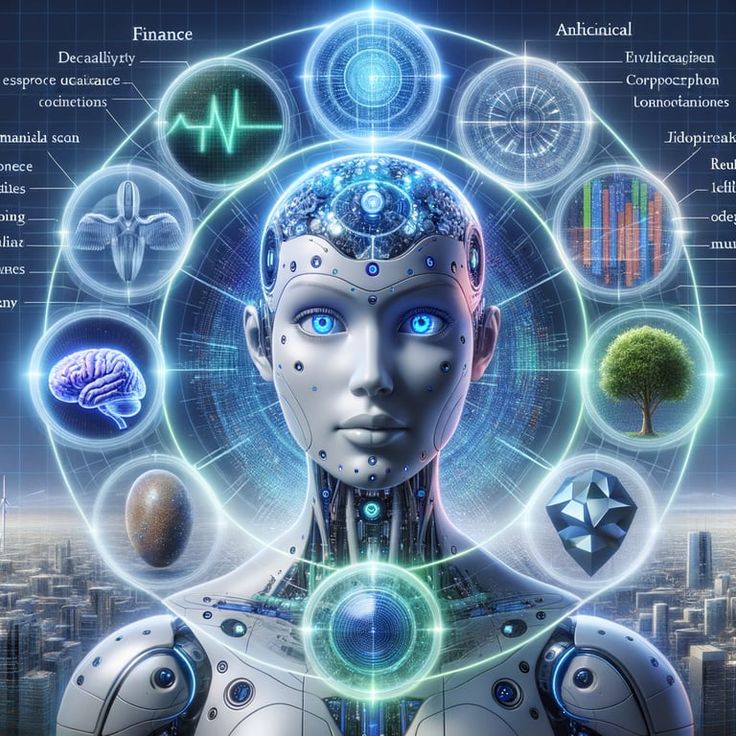
Artificial General Intelligence
Artificial general intelligence, or AGI, is all about creating computers that can think and learn like humans, allowing them to tackle any intellectual job. Unlike limited AI, which is great at specific tasks, AGI aims for true adaptability and versatility. To achieve AGI, we need to make significant strides in areas like machine learning, natural language understanding, and reasoning.
Researchers are still conducting studies on advanced neural networks, brain-inspired designs, and the moral implications of developing such systems, even though AGI is still only a theoretical idea. AGI has enormous potential ramifications that could redefine humanity’s relationship with technology.
In order to achieve AGI, issues like processing complexity, energy efficiency, and the compatibility of AI systems with human values must also be resolved.
What Does the Future Hold?
The future of AI has a lot of potential. Cutting-edge technologies like large-scale data analytics, sophisticated neural networks, and quantum computing are expected to fuel AI innovation. Applications in space exploration, healthcare, education, and climate science can revolutionize civilization and solve urgent global issues.
But the emergence of AI also brings up moral questions, such as those pertaining to privacy, job displacement, and biases in judgment. Maximizing AI’s advantages while reducing its threats will need responsible development and control. Navigating the benefits and problems of the AI-driven era will require cooperation between researchers, legislators, and business executives.
AI also has the ability to boost human ingenuity and creativity. AI can help people focus on higher-order problem-solving and creative expression by automating repetitive chores. Future generations’ readiness for an AI-driven environment will also be greatly aided by the incorporation of AI into education and skill development.